Cum hoc ergo propter hoc
- ncameron
- Apr 8, 2020
- 3 min read
Updated: Apr 9, 2020
A few days ago this graph kept popping up on social media:
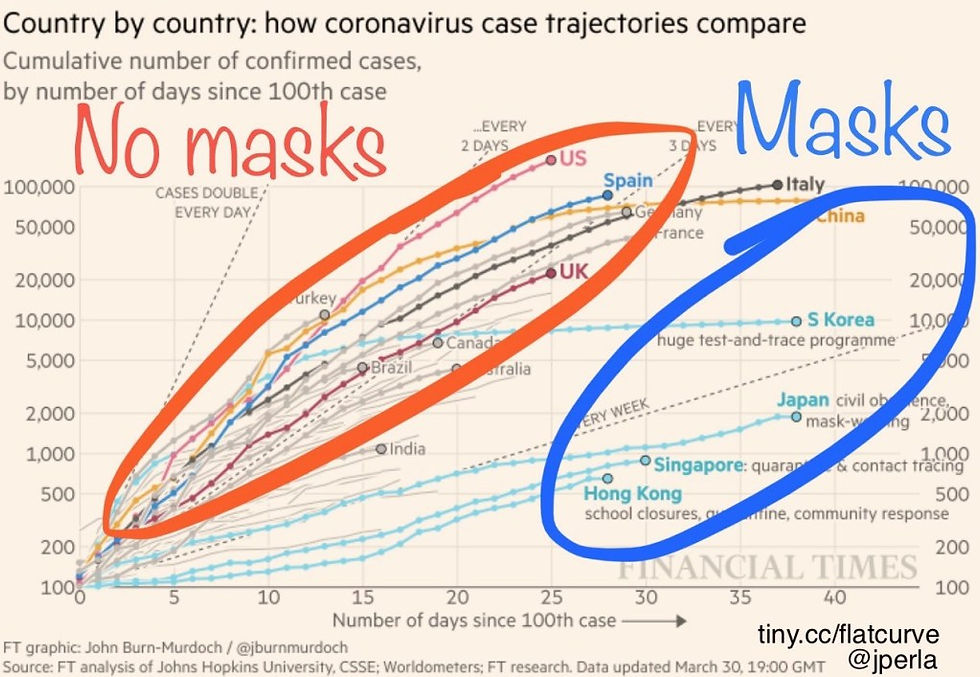
It is a graph originally taken from the Financial Times, and modified with orange and blue ink, by a Twitter user known as @jperla. It was used by @jperla, and many others since, to purport to 'demonstrate' or 'show' or 'prove', that the wearing of masks helps prevent the spread of coronavirus.
It does nothing of the sort, or course, because cum hoc ergo propter hoc.
As Wikipedia states; "In statistics, the phrase 'correlation does not imply causation' refers to the inability to legitimately deduce a cause-and-effect relationship between two variables solely on the basis of an observed association or correlation between them". This is the modern expression of the Latin phrase cum hoc ergo propter hoc - literally, 'with this, therefore because of this' (not to be confused with the other logical fallacy post hoc ergo propter hoc).
Furthermore, there are too many examples of tight correlations that can have no possible common causal link, for us to ignore. In fact, there is a whole web site devoted to them here. We shall illustrate with just one nice example:
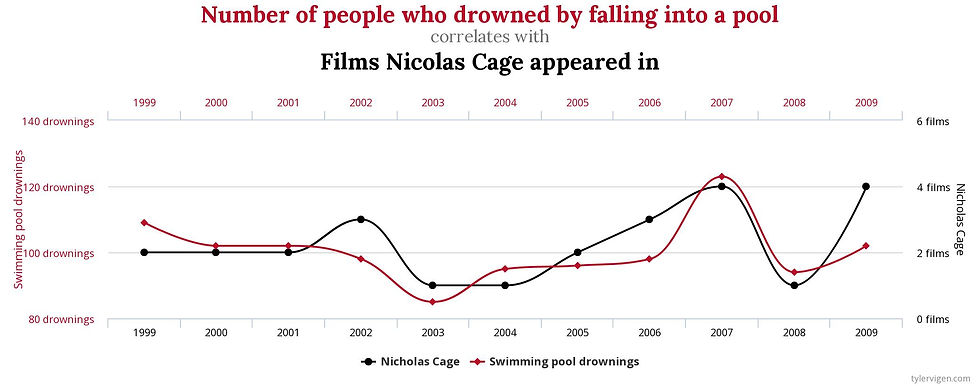
I said just one example, but i just can't resist this one:
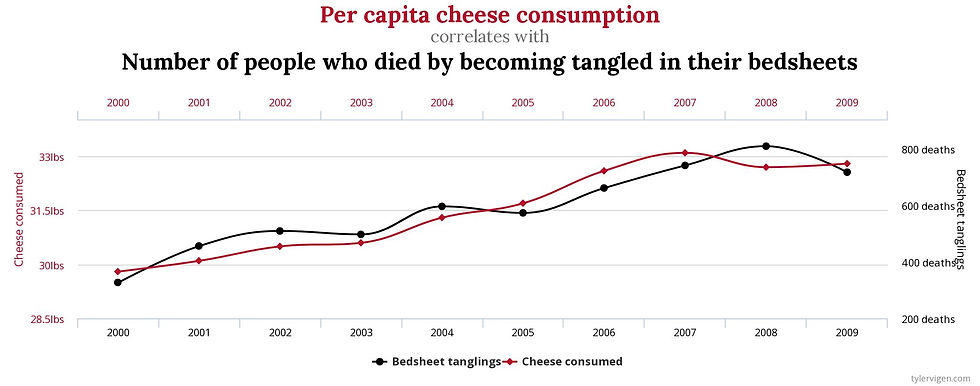
Mankind perpetually seeks to impose classification and order upon apparent chaos, and therefore, we often tend to jump to potentially useful but not necessarily true conclusions. If you were being chased by a lion in the Rift Valley 150,000 years ago, then jumping to conclusions may help save your life, even if some are erroneous. But that doesn't do for science.
Of course, it also depends on what you mean by the word 'imply'. One definition of imply is "that which strongly suggest the truth or existence of something not expressly stated". One could argue that a good correlation does mildly imply that there may be a causal relationship worth investigation - and that is fair enough. However, according to the 20th Century's clearest exponent of the scientific method, Karl Popper, in order to investigate such a potential causal relationship one should properly seek to prove that there isn't one - not that there is one. Proper scientific theory is characterised by being falsifiable.
For this reason I prefer to use the phrase 'correlation does not prove causation', which think is a better and more precise use of language.
In any event, where does all that leave us with this particular graph?
To repeat, I say that, beyond peradventure, it does not prove that masks can help prevent the spread of coronavirus. However, and it is a big however, it may yet be that masks do help prevent the spread of coronavirus. I merely state that his graph is not 'evidence' that they do, and this is because there are many other potential ways of explaining that graph. Such as (and some of these are even noted on the underlying chart) :
- the lock-down in some Asian countries was applied more speedily than in the West
- the Asian citizen may be more likely to obey government strictures
- some Asian governments may be more draconian in enforcing controls
- many Asian countries pursued broader testing regimes
- greater quarantine measures
- greater contact tracing.
There may, of course, also be another underlying causal factor, or combination of factors, of which we are yet unaware, or may never become aware - perhaps geographic, or based on the way that instances are recorded and measured and reported.
In conclusion though, I have to say that I do consider it somewhat strange that the WHO has consistently held the line that wearing a masks does not prevent wearers from getting it, nevertheless sufferers should wear masks, as they can help prevent wearers from spreading it.
Since when did such masks become unidirectional?
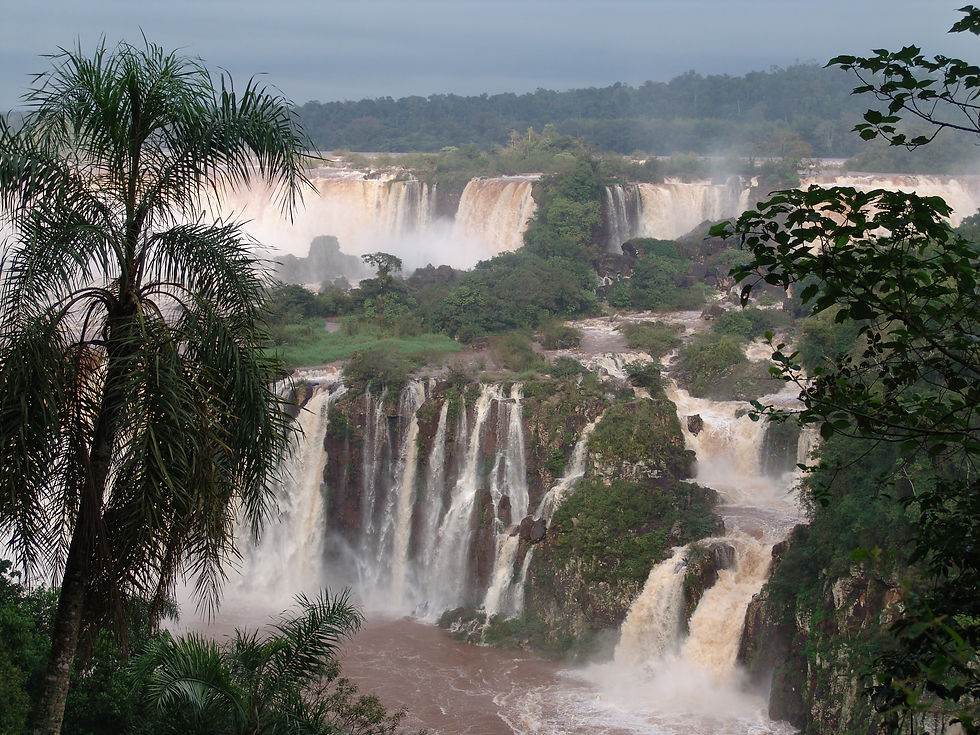
Comments